“Blessed is the nation that doesn’t need heroes" Goethe. “Hero-worship is strongest where there is least regard for human freedom.” Herbert Spencer. "My 80% friend isn't my 20% enemy" Ronald Reagan.
Search This Blog
Wednesday 13 July 2022
Sunday 10 July 2022
Friday 4 February 2022
Friday 28 January 2022
Sunday 16 January 2022
Will blockchain fulfil its democratic promise or will it become a tool of big tech?
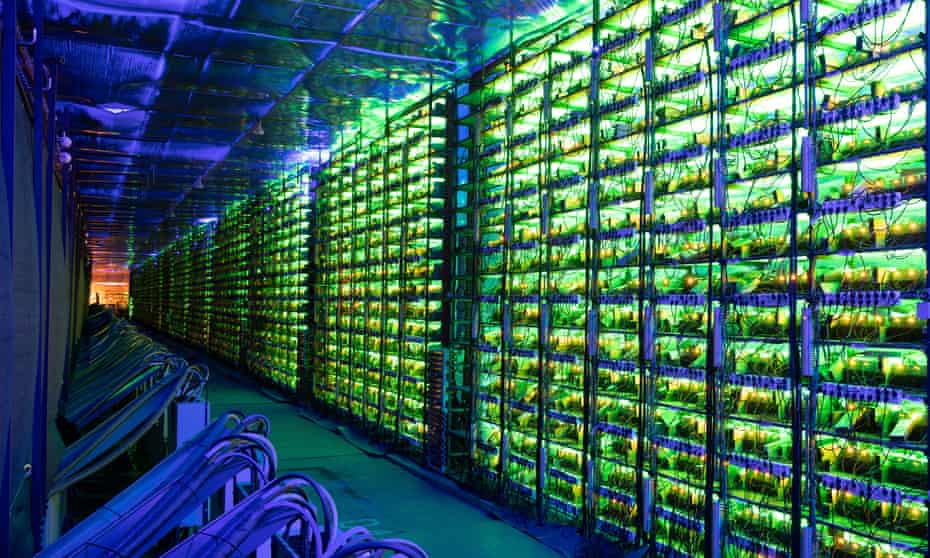
When the cryptocurrency bitcoin first made its appearance in 2009, an interesting divergence of opinions about it rapidly emerged. Journalists tended to regard it as some kind of incomprehensible money-laundering scam, while computer scientists, who were largely agnostic about bitcoin’s prospects, nevertheless thought that the distributed-ledger technology (the so-called blockchain) that underpinned the currency was a Big Idea that could have far-reaching consequences.
In this conviction they were joined by legions of techno-libertarians who viewed the technology as a way of enabling economic life without the oppressive oversight of central banks and other regulatory institutions. Blockchain technology had the potential to change the way we buy and sell, interact with government and verify the authenticity of everything from property titles to organic vegetables. It combined, burbled that well-known revolutionary body Goldman Sachs, “the openness of the internet with the security of cryptography to give everyone a faster, safer way to verify key information and establish trust”. Verily, cryptography would set us free.
At its core, a blockchain is just a ledger – a record of time-stamped transactions. These transactions can be any movement of money, goods or secure data – a purchase at a store, for example, the title to a piece of property, the assignment of an NHS number or a vaccination status, you name it. In the offline world, transactions are verified by some central third party – a government agency, a bank or Visa, say. But a blockchain is a distributed (ie, decentralised) ledger where verification (and therefore trustworthiness) comes not from a central authority but from a consensus of many users of the blockchain that a particular transaction is valid. Verified transactions are gathered into “blocks”, which are then “chained” together using heavy-duty cryptography so that, in principle, any attempt retrospectively to alter the details of a transaction would be visible. And oppressive, rent-seeking authorities such as Visa and Mastercard (or, for that matter, Stripe) are nowhere in the chain.
Given all that, it’s easy to see why the blockchain idea evokes utopian hopes: at last, technology is sticking it to the Man. In that sense, the excitement surrounding it reminds me of the early days of the internet, when we really believed that our contemporaries had invented a technology that was democratising and liberating and beyond the reach of established power structures. And indeed the network had – and still possesses – those desirable affordances. But we’re not using them to achieve their great potential. Instead, we’ve got YouTube and Netflix. What we underestimated, in our naivety, were the power of sovereign states, the ruthlessness and capacity of corporations and the passivity of consumers, a combination of which eventually led to corporate capture of the internet and the centralisation of digital power in the hands of a few giant corporations and national governments. In other words, the same entrapment as happened to the breakthrough communications technologies – telephone, broadcast radio and TV, and movies – in the 20th century, memorably chronicled by Tim Wu in his book The Master Switch.
Will this happen to blockchain technology? Hopefully not, but the enthusiastic endorsement of it by outfits such as Goldman Sachs is not exactly reassuring. The problem with digital technology is that, for engineers, it is both intrinsically fascinating and seductively challenging, which means that they acquire a kind of tunnel vision: they are so focused on finding solutions to the technical problems that they are blinded to the wider context. At the moment, for example, the consensus-establishing processes for verifying blockchain transactions requires intensive computation, with a correspondingly heavy carbon footprint. Reducing that poses intriguing technical challenges, but focusing on them means that the engineering community isn’t thinking about the governance issues raised by the technology. There may not be any central authority in a blockchain but, as Vili Lehdonvirta pointed out years ago, there are rules for what constitutes a consensus and, therefore, a question about who exactly sets those rules. The engineers? The owners of the biggest supercomputers on the chain? Goldman Sachs? These are ultimately political questions, not technical ones.
Blockchain engineers also don’t seem to be much interested in the needs of the humans who might ultimately be users of the technology. That, at any rate, is the conclusion that cryptographer Moxie Marlinspike came to in a fascinating examination of the technology. “When people talk about blockchains,” he writes, “they talk about distributed trust, leaderless consensus and all the mechanics of how that works, but often gloss over the reality that clients ultimately can’t participate in those mechanics. All the network diagrams are of servers, the trust model is between servers, everything is about servers. Blockchains are designed to be a network of peers, but not designed such that it’s really possible for your mobile device or your browser to be one of those peers.”
And we’re nowhere near that point yet.
Saturday 13 November 2021
Tuesday 24 August 2021
Wednesday 12 May 2021
Monday 10 May 2021
US-China rivalry drives the retreat of market economics
Gideon Rachman in The FT
Old ideas are like old clothes — wait long enough and they will come back into fashion. Thirty years ago, “industrial policy” was about as fashionable as a bowler hat. But now governments all over the world, from Washington to Beijing and New Delhi to London, are rediscovering the joy of subsidies and singing the praises of economic self-reliance and “strategic” investment.
The significance of this development goes well beyond economics. The international embrace of free markets and globalisation in the 1990s went hand in hand with declining geopolitical tension. The cold war was over and governments were competing to attract investment rather than to dominate territory.
Now the resurgence of geopolitical rivalry is driving the new fashion for state intervention in the economy. As trust declines between the US and China, so each has begun to see reliance on the other for any vital commodity — whether semiconductors or rare-earth minerals — as a dangerous vulnerability. Domestic production and security of supply are the new watchwords.
As the economic and industrial struggle intensifies, the US has banned the exports of key technologies to China and pushed to repatriate supply chains. It is also moving towards direct state-funding of semiconductor manufacturing. For its part, China has adopted a “dual circulation” economy policy that emphasises domestic demand and the achievement of “major breakthroughs in key technologies”. The government of Xi Jinping is also tightening state control over the tech sector.
The logic of an arms race is setting in, as each side justifies its moves towards protectionism as a response to actions by the other side. In Washington, the US-China Strategic Competition Act, currently wending its way through Congress, accuses China of pursuing “state-led mercantilist economic policies” and industrial espionage. The announcement in 2015 of Beijing’s “Made in China 2025” industrial strategy is often cited as a turning point. In Beijing, by contrast, it is argued that a fading America has turned against globalisation in an effort to block China’s rise. President Xi has said the backlash against globalisation in the west means China must become more self-reliant.
The new emphasis on industrial strategy is not confined to the US and China. In India, Narendra Modi’s government is promoting a policy of Atmanirbhar Bharat (self-reliant India), which encourages domestic production of key commodities. The EU published a paper on industrial strategy last year, which is seen as part of a drive towards strategic autonomy and less reliance on the outside world. Ursula von der Leyen, European Commission president, has called for Europe to have “mastery and ownership of key technologies”.
Even a Conservative administration in Britain is turning away from the laissez-faire economics championed by former prime minister Margaret Thatcher, and seeking to protect strategic industries. The government is reviewing whether to block the sale of Arm, a UK chipmaker, to Nvidia, a US company. The UK government has also bought a controlling stake in a failing satellite business, OneWeb.
Covid-19 has strengthened the fashion for industrial policy. The domestic production of vaccines is increasingly seen as a vital national interest. Even as they decry “vaccine nationalism” elsewhere, many governments have moved to restrict exports and to build up domestic suppliers. The lessons about national resilience learnt from the pandemic may now be applied to other areas, from energy to food supplies.
In the US, national security arguments for industrial policy are meshing with the wider backlash against globalisation and free trade. Joe Biden’s rhetoric is frankly protectionist. The president proclaimed to Congress: “All the investments in the American jobs plan will be guided by one principle: Buy American.”
In an article last year, Jake Sullivan, Mr Biden’s national security adviser, urged the security establishment to “move beyond the prevailing neoliberal economic philosophy of the past 40 years” and to accept that “industrial policy is deeply American”. The US, he argued, will continue to lose ground to China on key technologies such as 5G and solar panels, “if Washington continues to rely so heavily on private sector research and development”.
Many of these arguments will sound like common sense to voters. Protectionism and state intervention often does. But free-market economists are aghast. Swaminathan Aiyar, a prominent commentator in India, laments the return of the failed ideas of the past, arguing that: “Self sufficiency was what Nehru and Indira Gandhi tried in the 1960s and 1970s. It was a horrible and terrible flop.” Adam Posen, president of the Peterson Institute for International Economics in Washington, recently decried “America’s self-defeating economic retreat”, arguing that policies aimed at propping up chosen industries or regions usually end in costly failure.
As tensions rise between China, the US and other major powers, it is understandable that these countries will look at the security implications of key technologies. But claims by politicians that industrial policy will also produce better-paying jobs and a more productive economy deserve to be treated with deep scepticism. Sometimes ideas go out of fashion for a reason.
Thursday 22 April 2021
Burnt out: is the exhausting cult of productivity finally over?
In the US, they call it “hustle culture”: the idea that the ideal person for the modern age is one who is always on, always at work, always grafting. Your work is your life, and when you are not doing your hustle, you have a side-hustle. Like all the world’s worst ideas, it started in Silicon Valley, although it is a business-sector thing, rather than a California thing.
Since the earliest days of tech, the notion of “playbour”, work so enjoyable that it is interchangeable with leisure, has been the dream. From there, it spiralled in all directions: hobbies became something to monetise, wellness became a duty to your workplace and, most importantly, if you love your work, it follows that your colleagues are your intimates, your family.
Which is why an organisation such as Ustwo Games likes to call itself a “fampany”. “What the hell is that?” says Sarah Jaffe, author of Work Won’t Love You Back. “A lot of these companies’ websites use the word ‘family’, even though they have workers in Canada, workers in India, workers in the UK; a lot of us don’t even speak the same language and yet we’re a ‘family’.” Meanwhile, companies such as Facebook and Apple have offered egg-freezing to their employees, suggesting that you may have to defer having a real family if you work for a fake one.
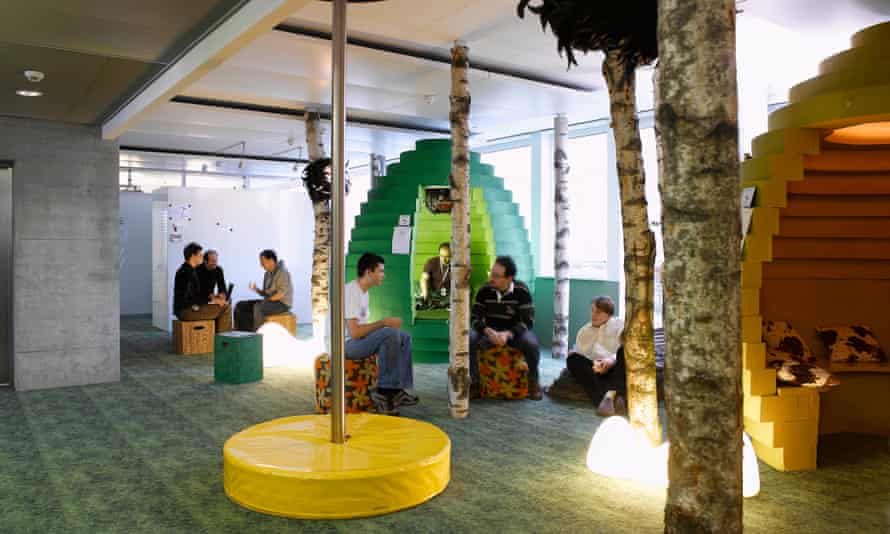
The tech companies’ attitudes have migrated into other “status” sectors, together with the workplaces that look like a kind of grownup soft-play, all colourful sofas and ping-pong and hot meals. In finance, food has become such a sign of pastoral care that Goldman Sachs recently sent junior employees hampers to make up for their 100-hour working weeks. If you actually cared about your staff, surely you would say it with proper working conditions, not fruit? But there is an even dicier subtext: when what you eat becomes your boss’s business, they are buying more than your time – they are buying your whole self.
Then Elon Musk weighed in to solve that niggling problem: what’s the point of it all? Making money for someone else, with your whole life? The billionaire reorientated the nature of work: it’s not a waypoint or distraction in the quest for meaning – work is meaning. “Nobody ever changed the world on 40 hours a week,” he memorably tweeted, concluding that people of vision worked 80 or more, eliding industry with passion, vision, society. Say what you like about him but he knows how to build a narrative.
Hustle culture has proved to be a durable and agile creed, changing its image and language while retaining its fundamentals. Sam Baker, author of The Shift: How I (Lost and) Found Myself After 40 – And You Can Too, worked an 80-hour week most of her life, editing magazines. “The 1980s were, ‘Put on a suit and work till you drop,’” she says. “Mark Zuckerberg is, ‘Put on a grey T-shirt and work till you drop.’” The difference, she says, is that “it’s all now cloaked in a higher mission”.
What has exposed the problems with this whole structure is the pandemic. It has wreaked some uncomfortable but helpful realisations – not least that the jobs with the least financial value are the ones we most rely on. Those sectors that Tim Jackson, professor of sustainable development at Surrey University and author of Post Growth: Life After Capitalism, describes as “chronically underinvested for so long, neglected for so long” and with “piss-poor” wages, are the ones that civilisation depends on: care work, retail, delivery.
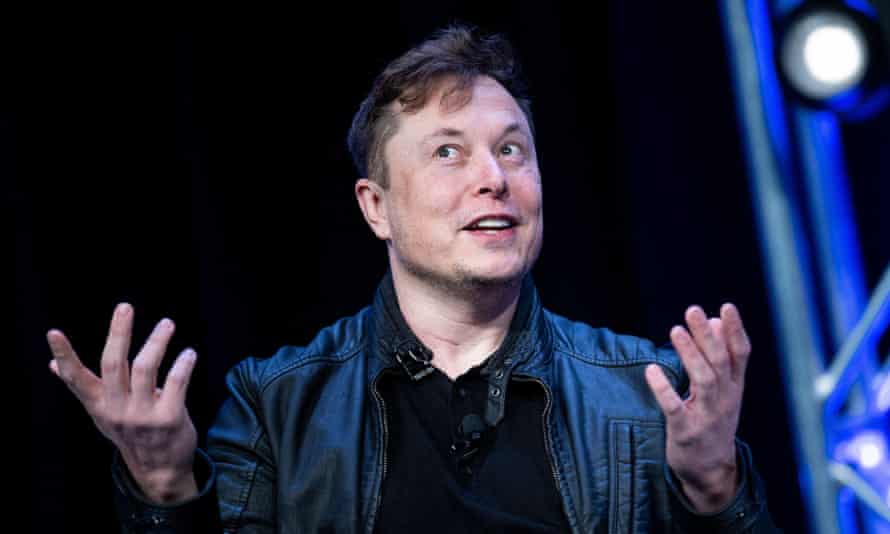
Many of the rest of us, meanwhile, have had to confront the nonessentiality of our jobs. Laura, 43, was working in private equity before the pandemic, but home working brought a realisation. Being apart from colleagues and only interacting remotely “distilled the job into the work rather than the emotions being part of something”. Not many jobs can take such harsh lighting. “It was all about making profit, and focusing on people who only care about the bottom line. I knew that. I’ve done it for 20-odd years. I just didn’t want to do it any more.”
Throw in some volunteering – which more than 12 million people have during the pandemic – and the scales dropped from her eyes. She ended up giving up her job to be a vaccination volunteer. She can afford to live on her savings for now, and as for what happens when the money runs out, she will cross that bridge when she comes to it. The four pillars of survival, on leaving work, are savings, spouses, downsizing and extreme thrift; generally speaking, people are happiest talking about the thrift and least happy talking about the savings.
Charlotte White, 47, had a similar revelation. She gave up a 20-plus-year career in advertising to volunteer at a food bank. “I felt so needed. This sounds very selfish but I have to admit that I’ve got a lot out of it. It’s the opposite of the advertising bullshit. I’d end each day thinking: ‘My God, I’ve really helped someone.’ I’ve lived in this neighbourhood for years, and there are all these people I’ve never met: older people, younger people, homeless people.”
With the spectre of mortality hovering insistently over every aspect of life, it is not surprising that people had their priorities upended. Neal, 50, lost his job as an accountant in January 2020. He started applying for jobs in the same field. “I was into three figures; my hit rate was something like one interview for 25. I think I was so uninterested that it was coming across in my application. I was pretending to be interested in spreadsheets and ledgers when thousands of people were dying, and it just did not sit right.” He is now working in a psychiatric intensive care unit, earning just above the minimum wage, and says: “I should have done it decades ago. I’m a much better support worker than I ever was an accountant.”
This is a constant motif: everybody mentions spreadsheets; everyone wishes they had made the change decades ago. “For nine months, my partner and I existed on universal credit,” Neal says, “and that was it. It was tough, we had to make adjustments, pay things later, smooth things out. But I thought: ‘If we can exist on that …’”
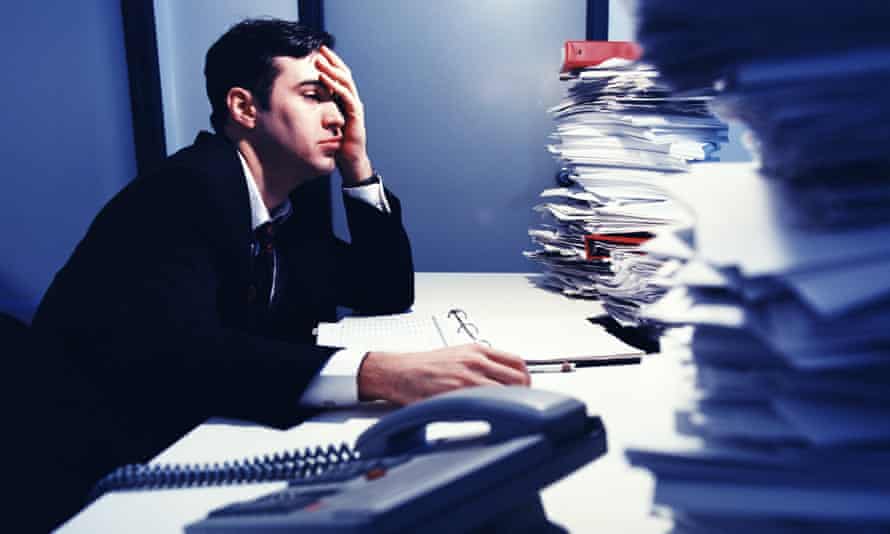
So why have we been swallowing these notions about work and value that were nonsense to begin with, and just getting sillier? We have known that the “higher mission” idea, whether it was emotional (being in a company that refers to itself as a “family”) or revolutionary (being “on” all the time in order to change the world) was, as Baker puts it “just fake, just another way of getting people to work 24 hours a day. It combined with the email culture, of always being available. I remember when I got my BlackBerry, I was working for Cosmopolitan, it was the best thing ever … It was only a matter of months before I was doing emails on holiday.”
But a lot of status came with feeling so indispensable. Unemployment is a famous driver of misery, and overemployment, to be so needed, can feel very bolstering. Many people describe having been anxious about the loss of status before they left their jobs; more anxious than about the money, where you can at least count what you are likely to have and plan around it. As Laura puts it, “not being on a ladder any more, not being in a race: there is something in life, you should always be moving forward, always going up”. And, when it came to it, other people didn’t see them as diminished.
Katherine Trebeck, of the Wellbeing Economy Alliance, is keen to broaden the focus of the productivity conversation. “To be able to have the choice, to design your own goals for your own life, to develop your own sense of where you get status and esteem is a huge privilege; there’s a socio-economic gradient associated with that level of autonomy,” she says. In other words: you have to have a certain level of financial security before your own emotional needs are at all relevant.
“When I was at Oxfam, we worked with young mothers experiencing poverty,” Trebeck says. “Just the pressure to shield their kids from looking poor made them skimp on the food they were providing. Society was forcing them to take those decisions between hunger and stigma.” She is sceptical about individual solutions and is much more focused on system change. Whether we are at the bottom or in the middle of this ladder, we are all part of the same story.
Part of the scam of the productivity narrative is to separate us, so that the “unskilled” are voiceless, discredited by their lack of skill, while the “skilled” don’t have anything to complain about because if they want to know what’s tough, they should try being unskilled. But in reality we are very interconnected – especially if working in the public sector – and you can burn out just by seeing too closely what is going on with other people.
Pam, 50, moved with her husband from London to the Peak District. They were both educationalists, he a headteacher, she in special educational needs (SEN). She describes what drove their decision: “If you think about a school, it’s a microcosm of life, and there have been very limited resources. Certainly in SEN, the lack of funding was desperate. Some kids just go through absolute hell: trying to get a CAMHS [Child and Adolescent Mental Health Services] appointment is nigh on impossible, kids have to be literally suicidal for someone to say: ‘OK, we’ll see you in two months.’”
They moved before the pandemic, and she found a part-time job with the National Trust, before lockdown forced a restructure. She hopes to resume working in the heritage sector when it reopens. Her husband still does some consultancy, but the bedrock of their security, financially speaking, is that the move out of London allowed them to “annihilate the mortgage”.
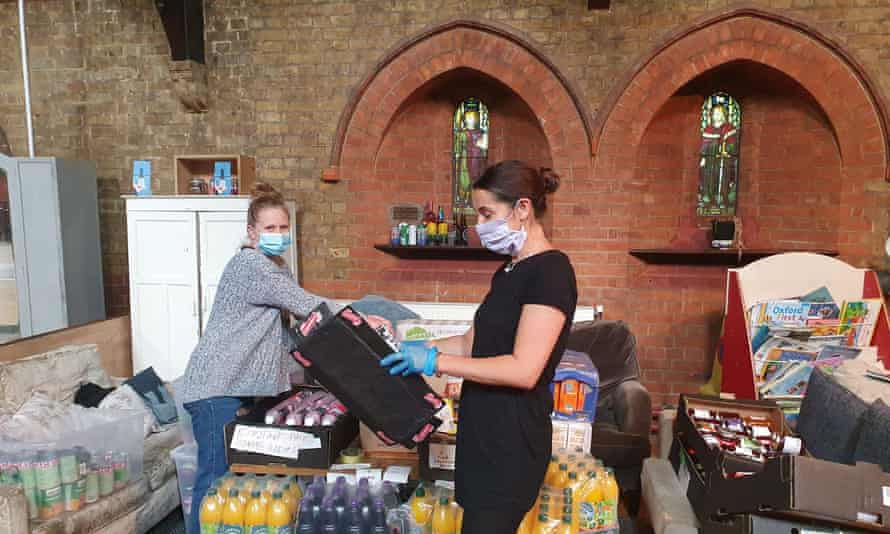
Creative and academic work, putatively so different from profit-driven sectors, nevertheless exploits its employees using, if anything, a heightened version of the same narrative: if what you do is who you are, then you’re incredibly lucky to be doing this thoughtful/artistic thing, and really, you should be paying us. Elizabeth, 39, was a performer, then worked in a theatre. “My eldest sister used to be an archaeologist, and that sounds different, but it’s the same: another job where they want you to be incredibly credentialed, incredibly passionate. But they still want to pay you minimum wage and God forbid you have a baby.”
There is also what the management consultants would call an opportunity cost, of letting work dominate your sense of who you are. You could go a whole life thinking your thing was maths, when actually it was empathy. I asked everyone if they had any regrets about their careerist years. Baker said: “Are you asking if I wish I’d had children? That’s what people usually mean when they ask that.” It actually wasn’t what I meant: whether you have children or not, the sense of what you have lost to hyperproductivity is more ineffable, that there was a better person inside you that never saw daylight.
When the furlough scheme came in, Jennifer, 39, an academic, leapt at the opportunity to cut her hours without sacrificing any pay. “I thought there’d be a stampede, but I was the only one.” She makes this elegant observation: “The difference between trying 110% and trying 80% is often not that big to other people.”
If the past year has made us rethink what skill means, upturn our notions of the value we bring to the world around us, fall out of love with our employers and question productivity in its every particular, as an individual goal as well as a social one, well, this, as the young people say, could be quite major. Certainly, I would like to see Elon Musk try to rebut this new consciousness in a tweet.
The European Super League is the perfect metaphor for global capitalism
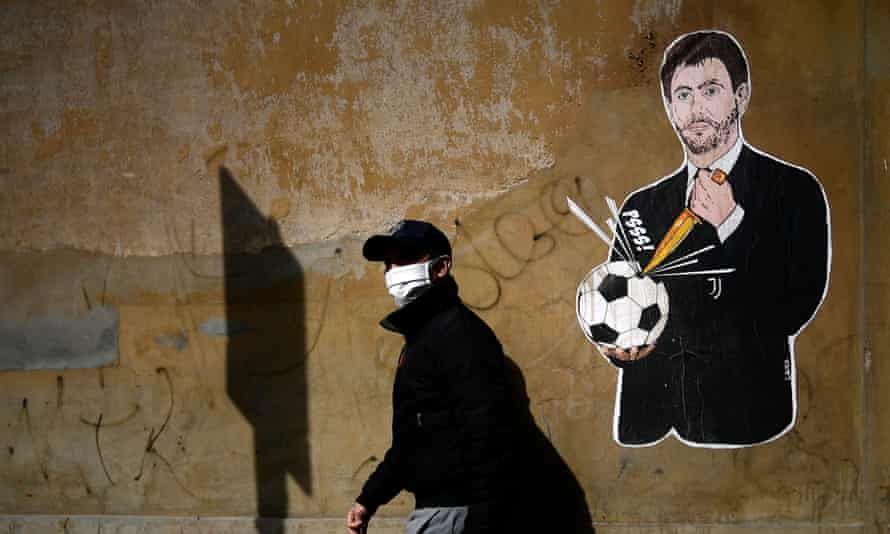
Back in the days of the Soviet Union, it was common to hear people on the left criticise the Kremlin for pursuing the wrong kind of socialism. There was nothing wrong with the theory, they said, rather the warped form of it conducted behind the iron curtain.
The same argument has surfaced this week amid the furious response to the now-aborted plans to form a European Super League for 20 football clubs, only this time from the right. Free-market purists say they hate the idea because it is the wrong form of capitalism.
They are both right and wrong about that. Free-market capitalism is supposed to work through competition, which means no barriers to entry for new, innovative products. In football’s case, that would be a go-ahead small club with a manager trying radical new training methods and fielding a crop of players it had nurtured itself or invested in through the transfer market. The league-winning Derby County and Nottingham Forest teams developed by Brian Clough in the 1970s would be an example of this.
Supporters of free-market capitalism say that the system can tolerate inequality provided there is the opportunity to better yourself. They are opposed to cartels and firms that use their market power to protect themselves from smaller and nimbler rivals. Nor do they like rentier capitalism, which is where people can make large returns from assets they happen to own but without doing anything themselves.
The organisers of the ESL have taken textbook free-market capitalism and turned it on its head. Having 15 of the 20 places guaranteed for the founder members represents a colossal barrier to entry and clearly stifles competition. There is not much chance of “creative destruction” if an elite group of clubs can entrench their position by trousering the bulk of the TV receipts that their matches will generate. Owners of the clubs are classic rentier capitalists.
Where the free-market critics of the ESL are wrong is in thinking the ESL is some sort of aberration, a one-off deviation from established practice, rather than a metaphor for what global capitalism has become: an edifice built on piles of debt where the owners of businesses say they love competition but do everything they can to avoid it. Just as the top European clubs have feeder teams that they can exploit for new talent, so the US tech giants have been busy buying up anything that looks like providing competition. It is why Google has bought a slew of rival online advertising vendors and why Facebook bought Instagram and WhatsApp.
For those who want to understand how the economics of football have changed, a good starting point is The Glory Game, a book Hunter Davies wrote about his life behind the scenes with Tottenham Hotspur, one of the wannabe members of the ESL, in the 1971-72 season. (Full disclosure: I am a Spurs season ticket holder.)
Davies’s book devotes a chapter to the directors of Spurs in the early 1970s, who were all lifelong supporters of the club and who received no payment for their services. They lived in Enfield, not in the Bahamas, which is where the current owner, Joe Lewis, resides as a tax exile. These were not radical men. They could not conceive of there ever being women on the board; they opposed advertising inside the ground and were only just coming round to the idea of a club shop to sell official Spurs merchandise. They were conservative in all senses of the word.
In the intervening half century, the men who made their money out of nuts and bolts and waste paper firms in north London have been replaced by oligarchs and hedge funds. TV, barely mentioned in the Glory Game, has arrived with its billions of pounds in revenue. Facilities have improved and the players are fitter, stronger and much better paid than those of the early 1970s. In very few sectors of modern Britain can it be said that the workers receive the full fruits of their labours: the Premier League is one of them.
Even so, the model is not really working and would have worked even less well had the ESL come about. And it goes a lot deeper than greed, something that can hardly be said to be new to football.
No question, greed is part of the story, because for some clubs the prospect of sharing an initial €3.5bn (£3bn) pot of money was just too tempting given their debts, but there was also a problem with the product on offer.
Some of the competitive verve has already been sucked out of football thanks to the concentration of wealth. In the 1970s, there was far more chance of a less prosperous club having their moment of glory: not only did Derby and Forest win the league, but Sunderland, Southampton and Ipswich won the FA Cup. Fans can accept the despair of defeat if they can occasionally hope for the thrill of victory, but the ESL was essentially a way for an elite to insulate itself against the risk of failure.
By presenting their half-baked idea in the way they did, the ESL clubs committed one of capitalism’s cardinal sins: they damaged their own brand. Companies – especially those that rely on loyalty to their product – do that at their peril, not least because it forces politicians to respond. Supporters have power and so do governments, if they choose to exercise it.
The ESL has demonstrated that global capitalism operates on the basis of rigged markets not free markets, and those running the show are only interested in entrenching existing inequalities. It was a truly bad idea, but by providing a lesson in economics to millions of fans it may have performed a public service.
Monday 22 March 2021
Thursday 18 March 2021
Saturday 2 May 2020
Deliveroo was the poster child for venture capitalism. It's not looking so good now
If any company can weather coronavirus well, it should be Deliveroo. The early days of lockdown saw demand surge for the service delivering food from restaurants and takeaways. The decision by several major restaurant and fast-food chains to shut for weeks during the early stages of lockdown might have dented demand, but as they begin to reopen for delivery – with most other activities still curtailed – prospects would seem bright for the tech company.
The reality looks quite different. Earlier this week, Deliveroo was reported to be cutting 367 jobs (and furloughing 50 more) from its workforce of 2,500. Others seem to be in similarly bleak positions – Uber is said to be discussing plans to let go around 20% of its workforce, some 5,400 roles. The broader UK start-up scene has asked for – and secured – government bailout funds.
Why Deliveroo is struggling during a crisis that should benefit its business model tells us about much more than just one start-up. The company’s nominal reasoning for needing cuts is that coronavirus will be followed by an economic downturn, which could hit orders. That’s plausible, but far from a given.
The financial crash of 2008 – which led to the most severe recession since the Great Depression – saw “cheap luxuries” perform quite well. People would swap a restaurant meal for, say, a £10 Marks & Spencer meal deal. Deliveroo is far cheaper than a restaurant meal for many people – there’s no need to pay for a childminder, or travel, and there’s no need to purchase alcohol at restaurant prices. Why would Deliveroo be so certain a downturn would be bad news?
The answer lies in the fact that Deliveroo’s real business model has almost nothing to do with making money from delivering food. Like pretty much every other start-up of its sort, once you take all of the costs into account, Deliveroo loses money on every single delivery it makes, even after taking a big cut from the restaurant and a delivery fee from the customer. Uber, now more than a decade old, still loses money for every ride its service offers and every meal its couriers deliver.
When every customer loses you money, it’s not good news for your business if customer numbers stay solid or even increase, unless there’s someone else who believes that’s a good thing. What these companies rely on is telling a story – largely to people who will invest in them. Their narrative is they’re “disrupting” existing industries, will build huge market share and customer bases, and thus can’t help but eventually become hugely profitable – just not yet.
This is the entire venture capital model – the financial model for Silicon Valley and the whole technology sector beyond it. Don’t worry about growing slowly and sustainably, don’t worry about profit, don’t worry about consequences. Just go flat out, hell for leather, and get as big as you can as fast as you can. It doesn’t matter than most companies will try and fail, provided a few succeed. Valuations will soar, the company will become publicly listed (a procedure known as an IPO) and then the company will either actually work out how to make profit – in which case, great – or by the time it’s clear it won’t, the venture capital funds have sold most of their stake at vast profits, and left regular investors holding the stock when the music stops.
This is a whole business model based on optimism. Without that optimism, and the accompanying free-flowing money to power through astronomical losses, the entire system breaks down. That’s the real struggle facing this type of company. It’s also why the very idea of bailing out this sector should be a joke: venture capital chases returns of at 10 times their investment, on the basis that it’s high risk and high reward. If we take out the element of “risk”, we’re basically just funnelling public money to make ultra-rich investors richer.
What pushes this beyond a tale that many of us might be happy to write down to karma, though, is the effects it has well beyond the rest of the world.
Tech giants move in on existing sectors that previously supported millions of jobs and helped people make their livelihoods – cabs and private hire, the restaurant business, to name just a few. They offer a new, subsidised alternative, that makes customers believe a service can be delivered much more cheaply, or that lets them cherry-pick from the restaurant experience – many restaurants relied on those alcohol sales with a meal to cover their margins, for example.
These start-ups come in to existing sectors essentially offering customers free money: £10 worth of stuff for a fiver. It turns out that’s easy to sell. But in the process, they rip the core out of existing businesses and reshape whole sectors of the economy in their image. And now, in the face of a pandemic, they are starting to struggle just like everyone else. It’s not hard to see how this sorry story ends. Having disrupted their industries to the point of leaving business after business on the verge of collapse, the start-ups could be tumbling down after them.
Thursday 19 March 2020
Can computers ever replace the classroom?
For a child prodigy, learning didn’t always come easily to Derek Haoyang Li. When he was three, his father – a famous educator and author – became so frustrated with his progress in Chinese that he vowed never to teach him again. “He kicked me from here to here,” Li told me, moving his arms wide.
Yet when Li began school, aged five, things began to click. Five years later, he was selected as one of only 10 students in his home province of Henan to learn to code. At 16, Li beat 15 million kids to first prize in the Chinese Mathematical Olympiad. Among the offers that came in from the country’s elite institutions, he decided on an experimental fast-track degree at Jiao Tong University in Shanghai. It would enable him to study maths, while also covering computer science, physics and psychology.
In his first year at university, Li was extremely shy. He came up with a personal algorithm for making friends in the canteen, weighing data on group size and conversation topic to optimise the chances of a positive encounter. The method helped him to make friends, so he developed others: how to master English, how to interpret dreams, how to find a girlfriend. While other students spent the long nights studying, Li started to think about how he could apply his algorithmic approach to business. When he graduated at the turn of the millennium, he decided that he would make his fortune in the field he knew best: education.
In person, Li, who is now 42, displays none of the awkwardness of his university days. A successful entrepreneur who helped create a billion-dollar tutoring company, Only Education, he is charismatic, and given to making bombastic statements. “Education is one of the industries that Chinese people can do much better than western people,” he told me when we met last year. The reason, he explained, is that “Chinese people are more sophisticated”, because they are raised in a society in which people rarely say what they mean.
Li is the founder of Squirrel AI, an education company that offers tutoring delivered in part by humans, but mostly by smart machines, which he says will transform education as we know it. All over the world, entrepreneurs are making similarly extravagant claims about the power of online learning – and more and more money is flowing their way. In Silicon Valley, companies like Knewton and Alt School have attempted to personalise learning via tablet computers. In India, Byju’s, a learning app valued at $6 billion, has secured backing from Facebook and the Chinese internet behemoth Tencent, and now sponsors the country’s cricket team. In Europe, the British company Century Tech has signed a deal to roll out an intelligent teaching and learning platform in 700 Belgian schools, and dozens more across the UK. Their promises are being put to the test by the coronavirus pandemic – with 849 million children worldwide, as of March 2020, shut out of school, we’re in the midst of an unprecedented experiment in the effectiveness of online learning.
But it’s in China, where President Xi Jinping has called for the nation to lead the world in AI innovation by 2030, that the fastest progress is being made. In 2018 alone, Li told me, 60 new AI companies entered China’s private education market. Squirrel AI is part of this new generation of education start-ups. The company has already enrolled 2 million student users, opened 2,600 learning centres in 700 cities across China, and raised $150m from investors. The company’s chief AI officer is Tom Mitchell, the former dean of computer science at Carnegie Mellon University, and its payroll also includes a roster of top Chinese talent, including dozens of “super-teachers” – an official designation given to the most expert teachers in the country. In January, during the worst of the outbreak, it partnered with the Shanghai education bureau to provide free products to students throughout the city.
Though the most ambitious features have yet to be built into Squirrel AI’s system, the company already claims to have achieved impressive results. At its HQ in Shanghai, I saw footage of downcast human teachers who had been defeated by computers in televised contests to see who could teach a class of students more maths in a single week. Experiments on the effectiveness of different types of teaching videos with test audiences have revealed that students learn more proficiently from a video presented by a good-looking young presenter than from an older expert teacher.
When we met, Li rhapsodised about a future in which technology will enable children to learn 10 or even 100 times more than they do today. Wild claims like these, typical of the hyperactive education technology sector, tend to prompt two different reactions. The first is: bullshit – teaching and learning is too complex, too human a craft to be taken over by robots. The second reaction is the one I had when I first met Li in London a year ago: oh no, the robot teachers are coming for education as we know it. There is some truth to both reactions, but the real story of AI education, it turns out, is a whole lot more complicated.
At a Squirrel AI learning centre high in an office building in Hangzhou, a city 70 miles west of Shanghai, a cursor jerked tentatively over the words “Modern technology has opened our eyes to many things”. Slouched at a hexagonal table in one of the centre’s dozen or so small classrooms, Huang Zerong, 14, was halfway through a 90-minute English tutoring session. As he worked through activities on his MacBook, a young woman with the kindly manner of an older sister sat next to him, observing his progress. Below, the trees of Xixi National Wetland Park barely stirred in the afternoon heat.
A question popped up on Huang’s screen, on which a virtual dashboard showed his current English level, unit score and learning focus – along with the sleek squirrel icon of Squirrel AI.
“India is famous for ________ industry.”
Huang read through the three possible answers, choosing to ignore “treasure” and “typical” and type “t-e-c-h-n-o-l-o-g-y” into the box.
“T____ is changing fast,” came the next prompt.
Huang looked towards the young woman, then he punched out “e-c-h-n-o-l-o-g-y” from memory. She clapped her hands together. “Good!” she said, as another prompt flashed up.
Huang had begun his English course, which would last for one term, a few months earlier with a diagnostic test. He had logged into the Squirrel AI platform on his laptop and answered a series of questions designed to evaluate his mastery of more than 10,000 “knowledge points” (such as the distinction between “belong to” and “belong in”). Based on his answers, Squirrel AI’s software had generated a precise “learning map” for him, which would determine which texts he would read, which videos he would see, which tests he would take.
As he worked his way through the course – with the occasional support of the human tutor by his side, or one of the hundreds accessible via video link from Squirrel AI’s headquarters in Shanghai – its contents were automatically updated, as the system perceived that Huang had mastered new knowledge.
Huang said he was less distracted at the learning centre than he was in school, and felt at home with the technology. “It’s fun,” he told me after class, eyes fixed on his lap. “It’s much easier to concentrate on the system because it’s a device.” His scores in English also seemed to be improving, which is why his mother had just paid the centre a further 91,000 RMB (about £11,000) for another year of sessions: two semesters and two holiday courses in each of four subjects, adding up to around 400 hours in total.
“Anyone can learn,” Li explained to me a few days later over dinner in Beijing. You just needed the right environment and the right method, he said.
The idea for Squirrel AI had come to him five years earlier. A decade at his tutoring company, Only Education, had left him frustrated. He had found that if you really wanted to improve a student’s progress, by far the best way was to find them a good teacher. But good teachers were rare, and turnover was high, with the best much in demand. Having to find and train 8,000 new teachers each year was limiting the amount students learned – and the growth of his business.
The answer, Li decided, was adaptive learning, where an intelligent computer-based system adjusts itself automatically to the best method for an individual learner. The idea of adaptive learning was not new, but Li was confident that developments in AI research meant that huge advances were now within reach. Rather than seeking to recreate the general intelligence of a human mind, researchers were getting impressive results by putting AI to work on specialised tasks. AI doctors are now equal to or better than humans at analysing X-rays for certain pathologies, while AI lawyers are carrying out legal research that would once have been done by clerks.
Following such breakthroughs, Li resolved to augment the efforts of his human teachers with a tireless, perfectly replicable virtual teacher. “Imagine a tutor who knows everything,” he told me, “and who knows everything about you.”
In Hangzhou, Huang was struggling with the word “hurry”. On his screen, a video appeared of a neatly groomed young teacher presenting a three-minute masterclass about how to use the word “hurry” and related phrases (“in a hurry” etc). Huang watched along.
Moments like these, where a short teaching input results in a small learning output, are known as “nuggets”. Li’s dream, which is the dream of adaptive education in general, is that AI will one day provide the perfect learning experience by ensuring that each of us get just the right chunk of content, delivered in the right way, at the right moment for our individual needs.
One way in which Squirrel AI improves its results is by constantly hoovering up data about its users. During Huang’s lesson, the system continuously tracked and recorded every one of his key strokes, cursor movements, right or wrong answers, texts read and videos watched. This data was time-stamped, to show where Huang had skipped over or lingered on a particular task. Each “nugget” (the video to watch or text to read) was then recommended to him based on an analysis of his data, accrued over hundreds of hours of work on Squirrel’s platform, and the data of 2 million other students. “Computer tutors can collect more teaching experience than a human would ever be able to collect, even in a hundred years of teaching,” Tom Mitchell, Squirrel AI’s chief AI officer, told me over the phone a few weeks later.
The speed and accuracy of Squirrel AI’s platform will depend, above all, on the number of student users it manages to sign up. More students equals more data. As each student works their way through a set of knowledge points, they leave a rich trail of information behind them. This data is then used to train the algorithms of the “thinking” part of the Squirrel AI system.
This is one reason why Squirrel AI has integrated its online business with bricks-and-mortar learning centres. Most children in China do not have access to laptops and high-speed internet. The learning centres mean the company can reach kids they otherwise would not be able to. One of the reasons Mitchell says he is glad to be working with Squirrel AI is the sheer volume of data that the company is gathering. “We’re going to have millions of natural examples,” he told me with excitement.
The dream of a perfect education delivered by machine is not new. For at least a century, generations of visionaries have predicted that the latest inventions will transform learning. “Motion pictures,” wrote the American inventor Thomas Edison in 1922, “are destined to revolutionise our schools.” The immersive power of movies would supposedly turbo-charge the learning process. Others made similar predictions for radio, television, computers and the internet. But despite small successes – the Open University, TV universities in China in the 1980s, or Khan Academy today, which reaches millions of students with its YouTube lessons – teachers have continued to teach, and learners to learn, in much the same way as before.
There are two reasons why today’s techno-evangelists are confident that AI can succeed where other technologies failed. First, they view AI not as a simple innovation but as a “general purpose technology” – that is, an epochal invention, like the printing press, which will fundamentally change the way we learn. Second, they believe its powers will shed new light on the working of the human brain – how repetitive practice grows expertise, for instance, or how interleaving (leaving gaps between learning different bits of material) can help us achieve mastery. As a result, we will be able to design adaptive algorithms to optimise the learning process.
UCL Institute of Education professor and machine learning expert Rose Luckin believes that one day we might see an AI-enabled “Fitbit for the mind” that would allow us to perceive in real-time what an individual knows, and how fast they are learning. The device would use sensors to gather data that forms a precise and ever-evolving map of a person’s abilities, which could be cross-referenced with insights into their motivational and nutritional state, say. This information would then be relayed to our minds, in real time, via a computer-brain interface. Facebook is already carrying out research in this field. Other firms are trialling eye tracking and helmets that monitor kids’ brainwaves.Get the Guardian’s award-winning long reads sent direct to you every Saturday morning
The supposed AI education revolution is not here yet, and it is likely that the majority of projects will collapse under the weight of their own hype. IBM’s adaptive tutor Knewton was pulled from US schools under pressure from parents concerned about their kids’ privacy, while Silicon Valley’s Alt School, launched to much fanfare in 2015 by a former Google executive, has burned through $174m of funding without landing on a workable business model. But global school closures owing to coronavirus may yet relax public attitudes to online learning – many online education companies are offering their products for free to all children out of school.
Daisy Christodoulou, a London-based education expert, suggests that too much time is spent speculating on what AI might one day do, rather than focusing on what it already can. It’s estimated that there are 900 million young people around the world today who aren’t currently on track to learn what they need to thrive. To help those kids, AI education doesn’t have to be perfect – it just needs to slightly improve on what they currently have.
In their book The Future of the Professions, Richard and Daniel Susskind argue that we tend to conceive of occupations as embodied in a person – a butcher or baker, doctor or teacher. As a result, we think of them as ‘too human’ to be taken over by machines. But to an algorithm, or someone designing one, a profession appears as something else: a long list of individual tasks, many of which may be mechanised. In education, that might be marking or motivating, lecturing or lesson planning. The Susskinds believe that where a machine can do any one of these tasks better and more cheaply than the average human, automation of that bit of the job is inevitable.
The point, in short, is that AI doesn’t have to match the general intelligence of humans to be useful – or indeed powerful. This is both the promise of AI, and the danger it poses. “People’s behaviour is already being manipulated,” Luckin cautioned. Devices that might one day enhance our minds are already proving useful in shaping them.
In May 2018, a group of students at Hangzhou’s Middle School No 11 returned to their classroom to find three cameras newly installed above the blackboard; they would now be under full-time surveillance in their lessons. “Previously when I had classes that I didn’t like very much, I would be lazy and maybe take naps,” a student told the local news, “but I don’t dare be distracted after the cameras were installed.” The head teacher explained that the system could read seven states of emotion on students’ faces: neutral, disgust, surprise, anger, fear, happiness and sadness. If the kids slacked, the teacher was alerted. “It’s like a pair of mystery eyes are constantly watching me,” the student told reporters.
The previous year, China’s state council had launched a plan for the role AI could play in the future of the country. Underpinning it were a set of beliefs: that AI can “harmonise” Chinese society; that for it to do so, the government should store data on every citizen; that companies, not the state, were best positioned to innovate; that no company should refuse access to the government to its data. In education, the paper called for new adaptive online learning systems powered by big data, and “all-encompassing ubiquitous intelligent environments” – or smart schools.
At AIAED, a conference in Beijing hosted by Squirrel AI, which I attended in May 2019, classroom surveillance was one of the most discussed topics – but the speakers tended to be more concerned about the technical question of how to optimise the effectiveness of facial and bodily monitoring technologies in the classroom, rather than the darker implications of collecting unprecedented amounts of data about children. These ethical questions are becoming increasingly important, with schools from India to the US currently trialling facial monitoring. In the UK, AI is being used today for things like monitoring student wellbeing, automating assessment and even in inspecting schools. Ben Williamson of the Centre for Research in Digital Education explains that this risks encoding biases or errors into the system and raises obvious privacy issues. “Now the school and university might be said to be studying their students too,” he told me.
While cameras in the classroom might outrage many parents in the UK or US, Lenora Chu, author of an influential book about the Chinese education system, argues that in China anything that improves a child’s learning tends to be viewed positively by parents. Squirrel AI even offers them the chance to watch footage of their child’s tutoring sessions. “There’s not that idea here that technology is bad,” said Chu, who moved from the US to Shanghai 10 years ago.
Rose Luckin suggested to me that a platform like Squirrel AI’s could one day mean an end to China’s notoriously punishing gaokao college entrance exam, which takes place for two days every June and largely determines a student’s education and employment prospects. If technology tracked a student throughout their school days, logging every keystroke, knowledge point and facial twitch, then the perfect record of their abilities on file could make such testing obsolete. Yet a system like this could also furnish the Chinese state – or a US tech company – with an eternal ledger of every step in a child’s development. It is not hard to imagine the grim uses to which this information could be put – for instance, if your behaviour in school was used to judge, or predict, your trustworthiness as an adult.
On the one hand, said Chu, the CCP wants to use AI to better prepare young people for the future economy, and to close the achievement gap between rural and urban schools. To this end, companies like Squirrel AI receive government support, such as access to prime office space in top business districts. At the same time, the CCP, as the state council put it, sees AI as “opportunity of the millennium” for “social construction”. That is, social control. The ability of AI to “grasp group cognition and psychological changes in a timely manner” through the surveillance of people’s movements, spending and other behaviours means it can play “an irreplaceable role in effectively maintaining social stability”.
The surveillance state is already penetrating deep into people’s lives. In 2019, there was a significant spike in China in the registration of patents for facial recognition and surveillance technology. All new mobile phones in China must now be registered via a facial scan. At the hotels I stayed in, Chinese citizens handed over their ID cards and checked in using face scanners. On the high-speed train to Beijing, the announcer repeatedly warned travellers to abide by the rules in order to maintain their personal credit. The notorious social credit system, which has been under trial in a handful of Chinese cities ahead of an expected nationwide roll out this year, awards or detracts points from an individual’s trustworthiness score, which affects their ability to travel and borrow money, among other things.
The result, explained Chu, is that all these interventions exert a subtle control over what people think and say. “You sense how the wind is blowing,” she told me. For the 12 million Muslim Uighurs in Xinjiang, however, that control is anything but subtle. Police checkpoints, complete with facial scanners, are ubiquitous. All mobile phones must have Jingwang (“clean net”) app installed, allowing the government to monitor your movements and browsing. Iris and fingerprint scans are required to access health services. As many as 1.5 million Uighurs, including children, have been interned at some point in a re-education camp in the interests of “harmony”.
As we shape the use of AI in education, it’s likely that AI will shape us too. Jiang Xueqin, an education researcher from Chengdu, is sceptical that it will be as revolutionary as proponents claim. “Parents are paying for a drug,” he told me over the phone. He thought tutoring companies such as New Oriental, TAL and Squirrel AI were simply preying on parents’ anxieties about their kids’ performance in exams, and only succeeding because test preparation was the easiest element of education to automate – a closed system with limited variables that allowed for optimisation. Jiang wasn’t impressed with the progress made, or the way that it engaged every child in a desperate race to conform to the measures of success imposed by the system.
One student I met at the learning centre in Hangzhou, Zhang Hen, seemed to have a deep desire to learn about the world – she told me how she loved Qu Yuan, a Tang dynasty romantic poet, and how she was a fan of Harry Potter – but that wasn’t the reason she was here. Her goal was much simpler: she had come to the centre to boost her test scores. That may seem disappointing to idealists who want education to offer so much more, but Zhang was realistic about the demands of the Chinese education system. She had tough exams that she needed to pass. A scripted system that helped her efficiently master the content of the high school entrance exam was exactly what she wanted.
On stage at AIAED, Tom Mitchell had presented a more ambitious vision for adaptive learning that went far beyond helping students cram for mindless tests. Much of what he was most excited by was possible only in theory, but his enthusiasm was palpable. As appealing as his optimism was, though, I felt unconvinced. It was clear that adaptive technologies might improve certain types of learning, but it was equally obvious that they might narrow the aims of education and provide new tools to restrict our freedom.
Li insists that one day his system will help all young people to flourish creatively. Though he allows that for now an expert human teacher still holds an edge over a robot, he is confident that AI will soon be good enough to evaluate and reply to students’ oral responses. In less than five years, Li imagines training Squirrel AI’s platform with a list of every conceivable question and every possible response, weighting an algorithm to favour those labelled “creative”. “That thing is very easy to do,” he said, “like tagging cats.”
For Li, learning has always been like that – like tagging cats. But there’s a growing consensus that our brains don’t work like computers. Whereas a machine must crunch through millions of images to be able to identify a cat as the collection of “features” that are present only in those images labelled “cat” (two triangular ears, four legs, two eyes, fur, etc), a human child can grasp the concept of “cat” from just a few real life examples, thanks to our innate ability to understand things symbolically. Where machines can’t compute meaning, our minds thrive on it. The adaptive advantage of our brains is that they learn continually through all of our senses by interacting with the environment, our culture and, above all, other people.
Li told me that even if AI fulfilled all of its promise, human teachers would still play a crucial role helping kids learn social skills. At Squirrel AI’s HQ, which occupies three floors of a gleaming tower next door to Microsoft and Mobike in Shanghai, I met some of the company’s young teachers. Each sat at a work console in a vast office space, headphones on, eyes focused on a laptop screen, their desks decorated with plastic pot plants and waving cats. As they monitored the dashboards of up to six students simultaneously, the face of a young learner would appear on the screen, asking for help, either via a chat box or through a video link. The teachers reminded me of workers in the gig economy, the Uber drivers of education. When I logged on to try out a Squirrel English lesson for myself, the experience was good, but my tutor seemed to be teaching to a script.
Squirrel AI’s head of communications, Joleen Liang, showed me photos from a recent trip she had taken to the remote mountains of Henan, to deliver laptops to disadvantaged students. Without access to the adaptive technology, their education would be a little worse. It was a reminder that Squirrel AI’s platform, like those of its competitors worldwide, doesn’t have to be better than the best human teachers – to improve people’s lives, it just needs to be good enough, at the right price, to supplement what we’ve got. The problem is that it is hard to see technology companies stopping there. For better and worse, their ambitions are bigger. “We could make a lot of geniuses,” Li told me.
Thursday 27 February 2020
Why your brain is not a computer
We are living through one of the greatest of scientific endeavours – the attempt to understand the most complex object in the universe, the brain. Scientists are accumulating vast amounts of data about structure and function in a huge array of brains, from the tiniest to our own. Tens of thousands of researchers are devoting massive amounts of time and energy to thinking about what brains do, and astonishing new technology is enabling us to both describe and manipulate that activity.
Every day, we hear about new discoveries that shed light on how brains work, along with the promise – or threat – of new technology that will enable us to do such far-fetched things as read minds, or detect criminals, or even be uploaded into a computer. Books are repeatedly produced that each claim to explain the brain in different ways.
And yet there is a growing conviction among some neuroscientists that our future path is not clear. It is hard to see where we should be going, apart from simply collecting more data or counting on the latest exciting experimental approach. As the German neuroscientist Olaf Sporns has put it: “Neuroscience still largely lacks organising principles or a theoretical framework for converting brain data into fundamental knowledge and understanding.” Despite the vast number of facts being accumulated, our understanding of the brain appears to be approaching an impasse.
I n 2017, the French neuroscientist Yves Frégnac focused on the current fashion of collecting massive amounts of data in expensive, large-scale projects and argued that the tsunami of data they are producing is leading to major bottlenecks in progress, partly because, as he put it pithily, “big data is not knowledge”.
“Only 20 to 30 years ago, neuroanatomical and neurophysiological information was relatively scarce, while understanding mind-related processes seemed within reach,” Frégnac wrote. “Nowadays, we are drowning in a flood of information. Paradoxically, all sense of global understanding is in acute danger of getting washed away. Each overcoming of technological barriers opens a Pandora’s box by revealing hidden variables, mechanisms and nonlinearities, adding new levels of complexity.”
The neuroscientists Anne Churchland and Larry Abbott have also emphasised our difficulties in interpreting the massive amount of data that is being produced by laboratories all over the world: “Obtaining deep understanding from this onslaught will require, in addition to the skilful and creative application of experimental technologies, substantial advances in data analysis methods and intense application of theoretic concepts and models.”
There are indeed theoretical approaches to brain function, including to the most mysterious thing the human brain can do – produce consciousness. But none of these frameworks are widely accepted, for none has yet passed the decisive test of experimental investigation. It is possible that repeated calls for more theory may be a pious hope. It can be argued that there is no possible single theory of brain function, not even in a worm, because a brain is not a single thing. (Scientists even find it difficult to come up with a precise definition of what a brain is.)
As observed by Francis Crick, the co-discoverer of the DNA double helix, the brain is an integrated, evolved structure with different bits of it appearing at different moments in evolution and adapted to solve different problems. Our current comprehension of how it all works is extremely partial – for example, most neuroscience sensory research has been focused on sight, not smell; smell is conceptually and technically more challenging. But the way that olfaction and vision work are different, both computationally and structurally. By focusing on vision, we have developed a very limited understanding of what the brain does and how it does it.
The nature of the brain – simultaneously integrated and composite – may mean that our future understanding will inevitably be fragmented and composed of different explanations for different parts. Churchland and Abbott spelled out the implication: “Global understanding, when it comes, will likely take the form of highly diverse panels loosely stitched together into a patchwork quilt.”
For more than half a century, all those highly diverse panels of patchwork we have been working on have been framed by thinking that brain processes involve something like those carried out in a computer. But that does not mean this metaphor will continue to be useful in the future. At the very beginning of the digital age, in 1951, the pioneer neuroscientist Karl Lashley argued against the use of any machine-based metaphor.
“Descartes was impressed by the hydraulic figures in the royal gardens, and developed a hydraulic theory of the action of the brain,” Lashley wrote. “We have since had telephone theories, electrical field theories and now theories based on computing machines and automatic rudders. I suggest we are more likely to find out about how the brain works by studying the brain itself, and the phenomena of behaviour, than by indulging in far-fetched physical analogies.”
This dismissal of metaphor has recently been taken even further by the French neuroscientist Romain Brette, who has challenged the most fundamental metaphor of brain function: coding. Since its inception in the 1920s, the idea of a neural code has come to dominate neuroscientific thinking – more than 11,000 papers on the topic have been published in the past 10 years. Brette’s fundamental criticism was that, in thinking about “code”, researchers inadvertently drift from a technical sense, in which there is a link between a stimulus and the activity of the neuron, to a representational sense, according to which neuronal codes represent that stimulus.
The unstated implication in most descriptions of neural coding is that the activity of neural networks is presented to an ideal observer or reader within the brain, often described as “downstream structures” that have access to the optimal way to decode the signals. But the ways in which such structures actually process those signals is unknown, and is rarely explicitly hypothesised, even in simple models of neural network function.
The processing of neural codes is generally seen as a series of linear steps – like a line of dominoes falling one after another. The brain, however, consists of highly complex neural networks that are interconnected, and which are linked to the outside world to effect action. Focusing on sets of sensory and processing neurons without linking these networks to the behaviour of the animal misses the point of all that processing.
By viewing the brain as a computer that passively responds to inputs and processes data, we forget that it is an active organ, part of a body that is intervening in the world, and which has an evolutionary past that has shaped its structure and function. This view of the brain has been outlined by the Hungarian neuroscientist György Buzsáki in his recent book The Brain from Inside Out. According to Buzsáki, the brain is not simply passively absorbing stimuli and representing them through a neural code, but rather is actively searching through alternative possibilities to test various options. His conclusion – following scientists going back to the 19th century – is that the brain does not represent information: it constructs it.
The metaphors of neuroscience – computers, coding, wiring diagrams and so on – are inevitably partial. That is the nature of metaphors, which have been intensely studied by philosophers of science and by scientists, as they seem to be so central to the way scientists think. But metaphors are also rich and allow insight and discovery. There will come a point when the understanding they allow will be outweighed by the limits they impose, but in the case of computational and representational metaphors of the brain, there is no agreement that such a moment has arrived. From a historical point of view, the very fact that this debate is taking place suggests that we may indeed be approaching the end of the computational metaphor. What is not clear, however, is what would replace it.
Scientists often get excited when they realise how their views have been shaped by the use of metaphor, and grasp that new analogies could alter how they understand their work, or even enable them to devise new experiments. Coming up with those new metaphors is challenging – most of those used in the past with regard to the brain have been related to new kinds of technology. This could imply that the appearance of new and insightful metaphors for the brain and how it functions hinges on future technological breakthroughs, on a par with hydraulic power, the telephone exchange or the computer. There is no sign of such a development; despite the latest buzzwords that zip about – blockchain, quantum supremacy (or quantum anything), nanotech and so on – it is unlikely that these fields will transform either technology or our view of what brains do.
One sign that our metaphors may be losing their explanatory power is the widespread assumption that much of what nervous systems do, from simple systems right up to the appearance of consciousness in humans, can only be explained as emergent properties – things that you cannot predict from an analysis of the components, but which emerge as the system functions.
In 1981, the British psychologist Richard Gregory argued that the reliance on emergence as a way of explaining brain function indicated a problem with the theoretical framework: “The appearance of ‘emergence’ may well be a sign that a more general (or at least different) conceptual scheme is needed … It is the role of good theories to remove the appearance of emergence. (So explanations in terms of emergence are bogus.)”
This overlooks the fact that there are different kinds of emergence: weak and strong. Weak emergent features, such as the movement of a shoal of tiny fish in response to a shark, can be understood in terms of the rules that govern the behaviour of their component parts. In such cases, apparently mysterious group behaviours are based on the behaviour of individuals, each of which is responding to factors such as the movement of a neighbour, or external stimuli such as the approach of a predator.
This kind of weak emergence cannot explain the activity of even the simplest nervous systems, never mind the working of your brain, so we fall back on strong emergence, where the phenomenon that emerges cannot be explained by the activity of the individual components. You and the page you are reading this on are both made of atoms, but your ability to read and understand comes from features that emerge through atoms in your body forming higher-level structures, such as neurons and their patterns of firing – not simply from atoms interacting.
Strong emergence has recently been criticised by some neuroscientists as risking “metaphysical implausibility”, because there is no evident causal mechanism, nor any single explanation, of how emergence occurs. Like Gregory, these critics claim that the reliance on emergence to explain complex phenomena suggests that neuroscience is at a key historical juncture, similar to that which saw the slow transformation of alchemy into chemistry. But faced with the mysteries of neuroscience, emergence is often our only resort. And it is not so daft – the amazing properties of deep-learning programmes, which at root cannot be explained by the people who design them, are essentially emergent properties.
Interestingly, while some neuroscientists are discombobulated by the metaphysics of emergence, researchers in artificial intelligence revel in the idea, believing that the sheer complexity of modern computers, or of their interconnectedness through the internet, will lead to what is dramatically known as the singularity. Machines will become conscious.
There are plenty of fictional explorations of this possibility (in which things often end badly for all concerned), and the subject certainly excites the public’s imagination, but there is no reason, beyond our ignorance of how consciousness works, to suppose that it will happen in the near future. In principle, it must be possible, because the working hypothesis is that mind is a product of matter, which we should therefore be able to mimic in a device. But the scale of complexity of even the simplest brains dwarfs any machine we can currently envisage. For decades – centuries – to come, the singularity will be the stuff of science fiction, not science.Get the Guardian’s award-winning long reads sent direct to you every Saturday morning
A related view of the nature of consciousness turns the brain-as-computer metaphor into a strict analogy. Some researchers view the mind as a kind of operating system that is implemented on neural hardware, with the implication that our minds, seen as a particular computational state, could be uploaded on to some device or into another brain. In the way this is generally presented, this is wrong, or at best hopelessly naive.
The materialist working hypothesis is that brains and minds, in humans and maggots and everything else, are identical. Neurons and the processes they support – including consciousness – are the same thing. In a computer, software and hardware are separate; however, our brains and our minds consist of what can best be described as wetware, in which what is happening and where it is happening are completely intertwined.
Imagining that we can repurpose our nervous system to run different programmes, or upload our mind to a server, might sound scientific, but lurking behind this idea is a non-materialist view going back to Descartes and beyond. It implies that our minds are somehow floating about in our brains, and could be transferred into a different head or replaced by another mind. It would be possible to give this idea a veneer of scientific respectability by posing it in terms of reading the state of a set of neurons and writing that to a new substrate, organic or artificial.
But to even begin to imagine how that might work in practice, we would need both an understanding of neuronal function that is far beyond anything we can currently envisage, and would require unimaginably vast computational power and a simulation that precisely mimicked the structure of the brain in question. For this to be possible even in principle, we would first need to be able to fully model the activity of a nervous system capable of holding a single state, never mind a thought. We are so far away from taking this first step that the possibility of uploading your mind can be dismissed as a fantasy, at least until the far future.
For the moment, the brain-as-computer metaphor retains its dominance, although there is disagreement about how strong a metaphor it is. In 2015, the roboticist Rodney Brooks chose the computational metaphor of the brain as his pet hate in his contribution to a collection of essays entitled This Idea Must Die. Less dramatically, but drawing similar conclusions, two decades earlier the historian S Ryan Johansson argued that “endlessly debating the truth or falsity of a metaphor like ‘the brain is a computer’ is a waste of time. The relationship proposed is metaphorical, and it is ordering us to do something, not trying to tell us the truth.”
On the other hand, the US expert in artificial intelligence, Gary Marcus, has made a robust defence of the computer metaphor: “Computers are, in a nutshell, systematic architectures that take inputs, encode and manipulate information, and transform their inputs into outputs. Brains are, so far as we can tell, exactly that. The real question isn’t whether the brain is an information processor, per se, but rather how do brains store and encode information, and what operations do they perform over that information, once it is encoded.”
Marcus went on to argue that the task of neuroscience is to “reverse engineer” the brain, much as one might study a computer, examining its components and their interconnections to decipher how it works. This suggestion has been around for some time. In 1989, Crick recognised its attractiveness, but felt it would fail, because of the brain’s complex and messy evolutionary history – he dramatically claimed it would be like trying to reverse engineer a piece of “alien technology”. Attempts to find an overall explanation of how the brain works that flow logically from its structure would be doomed to failure, he argued, because the starting point is almost certainly wrong – there is no overall logic.
Reverse engineering a computer is often used as a thought experiment to show how, in principle, we might understand the brain. Inevitably, these thought experiments are successful, encouraging us to pursue this way of understanding the squishy organs in our heads. But in 2017, a pair of neuroscientists decided to actually do the experiment on a real computer chip, which had a real logic and real components with clearly designed functions. Things did not go as expected.
The duo – Eric Jonas and Konrad Paul Kording – employed the very techniques they normally used to analyse the brain and applied them to the MOS 6507 processor found in computers from the late 70s and early 80s that enabled those machines to run video games such as Donkey Kong and Space Invaders.
First, they obtained the connectome of the chip by scanning the 3510 enhancement-mode transistors it contained and simulating the device on a modern computer (including running the games programmes for 10 seconds). They then used the full range of neuroscientific techniques, such as “lesions” (removing transistors from the simulation), analysing the “spiking” activity of the virtual transistors and studying their connectivity, observing the effect of various manipulations on the behaviour of the system, as measured by its ability to launch each of the games.
Despite deploying this powerful analytical armoury, and despite the fact that there is a clear explanation for how the chip works (it has “ground truth”, in technospeak), the study failed to detect the hierarchy of information processing that occurs inside the chip. As Jonas and Kording put it, the techniques fell short of producing “a meaningful understanding”. Their conclusion was bleak: “Ultimately, the problem is not that neuroscientists could not understand a microprocessor, the problem is that they would not understand it given the approaches they are currently taking.”
This sobering outcome suggests that, despite the attractiveness of the computer metaphor and the fact that brains do indeed process information and somehow represent the external world, we still need to make significant theoretical breakthroughs in order to make progress. Even if our brains were designed along logical lines, which they are not, our present conceptual and analytical tools would be completely inadequate for the task of explaining them. This does not mean that simulation projects are pointless – by modelling (or simulating) we can test hypotheses and, by linking the model with well-established systems that can be precisely manipulated, we can gain insight into how real brains function. This is an extremely powerful tool, but a degree of modesty is required when it comes to the claims that are made for such studies, and realism is needed with regard to the difficulties of drawing parallels between brains and artificial systems.
Even something as apparently straightforward as working out the storage capacity of a brain falls apart when it is attempted. Such calculations are fraught with conceptual and practical difficulties. Brains are natural, evolved phenomena, not digital devices. Although it is often argued that particular functions are tightly localised in the brain, as they are in a machine, this certainty has been repeatedly challenged by new neuroanatomical discoveries of unsuspected connections between brain regions, or amazing examples of plasticity, in which people can function normally without bits of the brain that are supposedly devoted to particular behaviours.
In reality, the very structures of a brain and a computer are completely different. In 2006, Larry Abbott wrote an essay titled “Where are the switches on this thing?”, in which he explored the potential biophysical bases of that most elementary component of an electronic device – a switch. Although inhibitory synapses can change the flow of activity by rendering a downstream neuron unresponsive, such interactions are relatively rare in the brain.
A neuron is not like a binary switch that can be turned on or off, forming a wiring diagram. Instead, neurons respond in an analogue way, changing their activity in response to changes in stimulation. The nervous system alters its working by changes in the patterns of activation in networks of cells composed of large numbers of units; it is these networks that channel, shift and shunt activity. Unlike any device we have yet envisaged, the nodes of these networks are not stable points like transistors or valves, but sets of neurons – hundreds, thousands, tens of thousands strong – that can respond consistently as a network over time, even if the component cells show inconsistent behaviour.
Understanding even the simplest of such networks is currently beyond our grasp. Eve Marder, a neuroscientist at Brandeis University, has spent much of her career trying to understand how a few dozen neurons in the lobster’s stomach produce a rhythmic grinding. Despite vast amounts of effort and ingenuity, we still cannot predict the effect of changing one component in this tiny network that is not even a simple brain.
This is the great problem we have to solve. On the one hand, brains are made of neurons and other cells, which interact together in networks, the activity of which is influenced not only by synaptic activity, but also by various factors such as neuromodulators. On the other hand, it is clear that brain function involves complex dynamic patterns of neuronal activity at a population level. Finding the link between these two levels of analysis will be a challenge for much of the rest of the century, I suspect. And the prospect of properly understanding what is happening in cases of mental illness is even further away.
Not all neuroscientists are pessimistic – some confidently claim that the application of new mathematical methods will enable us to understand the myriad interconnections in the human brain. Others – like myself – favour studying animals at the other end of the scale, focusing our attention on the tiny brains of worms or maggots and employing the well-established approach of seeking to understand how a simple system works and then applying those lessons to more complex cases. Many neuroscientists, if they think about the problem at all, simply consider that progress will inevitably be piecemeal and slow, because there is no grand unified theory of the brain lurking around the corner.
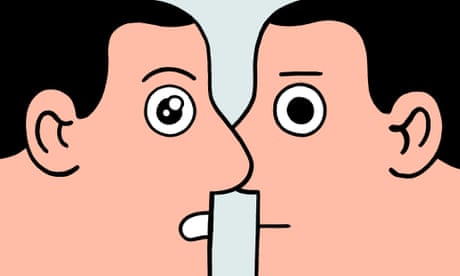
There are many alternative scenarios about how the future of our understanding of the brain could play out: perhaps the various computational projects will come good and theoreticians will crack the functioning of all brains, or the connectomes will reveal principles of brain function that are currently hidden from us. Or a theory will somehow pop out of the vast amounts of imaging data we are generating. Or we will slowly piece together a theory (or theories) out of a series of separate but satisfactory explanations. Or by focusing on simple neural network principles we will understand higher-level organisation. Or some radical new approach integrating physiology and biochemistry and anatomy will shed decisive light on what is going on. Or new comparative evolutionary studies will show how other animals are conscious and provide insight into the functioning of our own brains. Or unimagined new technology will change all our views by providing a radical new metaphor for the brain. Or our computer systems will provide us with alarming new insight by becoming conscious. Or a new framework will emerge from cybernetics, control theory, complexity and dynamical systems theory, semantics and semiotics. Or we will accept that there is no theory to be found because brains have no overall logic, just adequate explanations of each tiny part, and we will have to be satisfied with that.